The role of AI in software testing automation
In short, the role of AI tools for test automation is doing things at a scale that humans canāt achieve:
- AI solutions make QA faster
- AI solutions make QA more precise
- AI solutions make QA innovative
That last point is perhaps the most future-looking one. Although we still live in the world of limited AI that canāt really āthinkā for itself, it can very much amplify preassigned concepts. As part of the test automation tool functionality within aqua, we have been looking at auto-creating new tests without human input. Early results are quite promising if there is a good database of tests for AI to draw inspiration from, and we canāt wait to launch a public beta.
Enterprise-grade TMS with AI test generation
Benefits of using AI tools for QA manager
Letās list the key advantages of looking past the buzzword stigma of AI automation testing tools:Ā
- AI test automation tools bring tangible efficiency gains. Running automated tests does not really require AI functionality, but your test managerās automation suite can be optimised.
- When testing our own solution, it usually takes us about 30 minutes to do a full run of all automated tests. At some point, the only way to reduce this time is to remove some tests. You would normally take out tests that fail rarely and/or are too flaky to get definitive results. Identifying such tests is where AI for QA comes into play.
- Grouping tests is another angle of optimising your test suite with artificial intelligence. Even if you donāt have inconclusive or rarely failing tests, there are cases where you can still avoid running all of them. Deploying a hotfix usually means running regression testing as the first priority, while tests that usually reveal minor bugs can be brushed aside.
- Similarly, you can bundle user interface tests and run them when you have the time to do so. There are cases when releasing a new feature faster is more important than making the visuals pixel-perfect. In fact, it happens all the time in B2C markets.
- Smart work allocation is another benefit of AI solutions. We are not talking about something as simple as creating a ticket in your bug reporting tool. Letās go back to the UI testing example from earlier. When time is of the essence, your devs may not even have the time to address element placement issues that a test found. This is where an AI-powered tool can assess the difficulty of a bug fix, tap into your issue management software to see the priority of pending tasks for all devs, and then slot a bug fix into the schedule of the right person.
- Even without scheduling, it is extremely beneficial to have your software assess the severity of discovered bugs. It would be truly amazing to have the machine rank bugs based on their impact on the bottom line of a business. Sadly this is not widely available in modern AI testing tools, open-source or otherwise.
- Time management is another area where AI could help a lot. We have asked a number of QA specialists to share their time estimation techniques, but there is no one-size-fits-all solution. This is where artificial intelligence can try to chip in and potentially create more chaos to help you achieve balance.
- One exciting application that I see is using AI for poker planning. The analysis of similar tasks can be the tiebreaker for team members who can’t convince their colleagues to agree with their higher or lower estimate. Actually, AI could look at everyoneās votes across a few planning sessions to see whose estimates have been more or less accurate than the actual effort. This data can be used for some sort of a multiplier or again applied for tie breaking purposes.
We cover some more benefits in our overview of AI testing trends. This is a practical, up-to-date look into applying AI to quality assurance with extra emphasis on GPT-powered solutions.
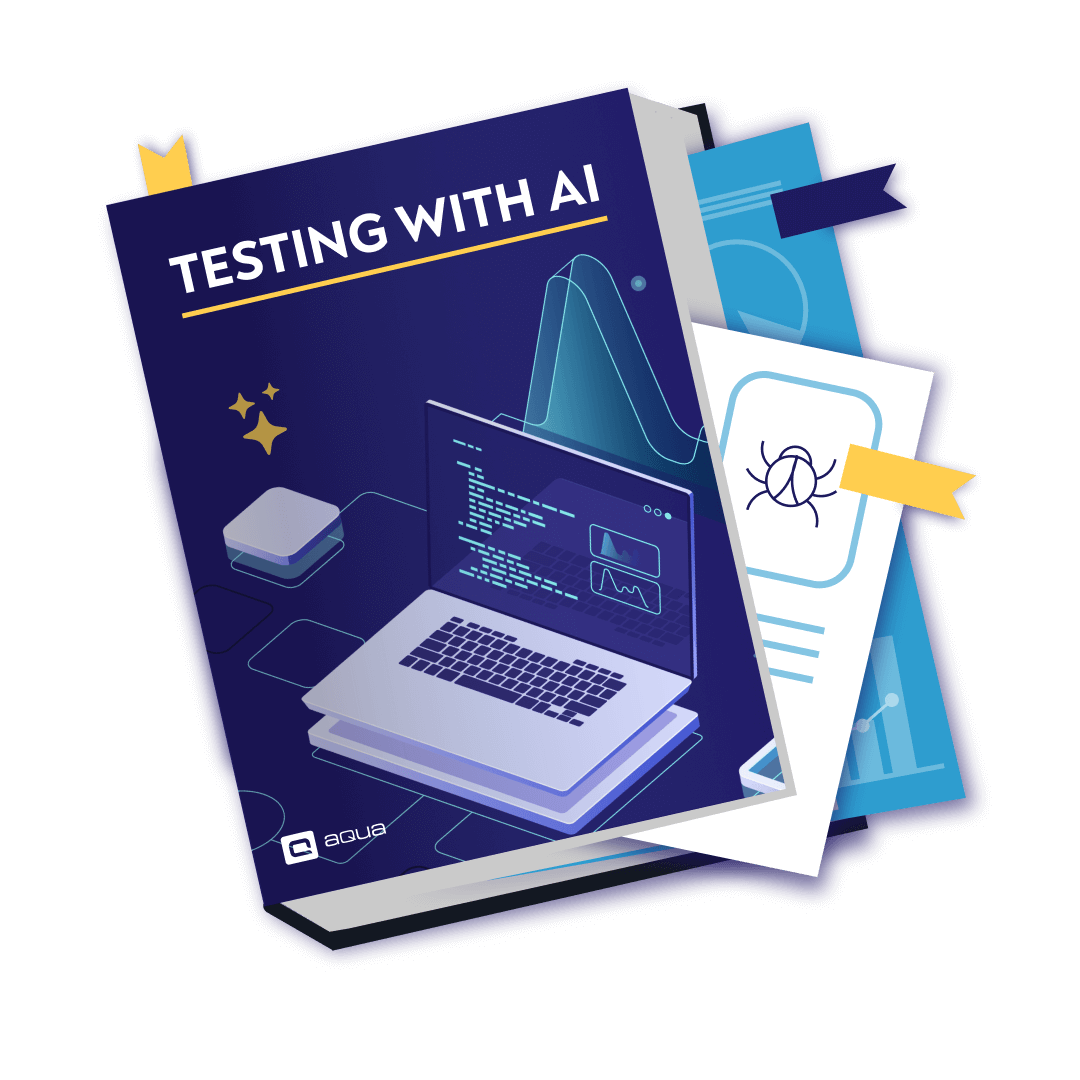
Learn the 5 AI testing trends to save 12.8 hrs/week per specialist
Pitfalls of using AI for software testing
Here are potential issues that you need to keep in mind before committing to testing with AI.
- AI solutions can be hard to validate. AI-focused tools are too fresh to have a meaningful number of reviews. Traditional players will mostly have reviews that were written before the vendor introduced AI functionality. They also happen to have a less accessible and sometimes gimped trial offering compared to up-and-coming companies.
Then there is a separate issue of knowing if your potential vendor actually uses AI. In 2019, a venture capitalist investor manually reviewed 2,830 āAIā companies in Europe and found out that 40% did not actually have such tech. Some go as far as outright admitting there is no AI in their work.
'You simulate what the ultimate experience of something is going to be. And a lot of time when it comes to AI, there is a person behind the curtain rather than an algorithm'.
Long story short, there is quite some truth to this tongue-in-cheek tweet from a few years back.
How to start an AI startup
1. Hire a bunch of minimum wage humans to pretend to be AI pretending to be human
2. Wait for AI to be invented
— Gregory Koberger (@gkoberger) March 1, 2016
- AI solutions are not a silver bullet. You will still at the very least need a senior specialist reviewing AI-generated tests and/or prioritisation to see if they are sane. You will still need a human to verify that there is a measurable improvement (or at least no degradation) from following AI recommendations. Then there are solutions that invite more human involvement to make better automated suggestions. These days, AI is the tool to amplify expertise, not replace it.Ā
- AI solutions require commitment on both sides. Ultimately, you will need to either help the vendor tune their AI with your input or learn to work around the quirks of a less interactive implementation. This is time and money that you could have invested in traditional test automation among other things. But what if the promising AI quality assurance startup that you picked ends up missing or losing VC funding? What if a conservative player does not like how their AI beta works out and pulls the plug? Do your research and make sure you donāt get more excited and confident than your vendor is.
Future of AI in Testing Automation
The future of AI in testing automation is filled with potential, particularly in the automated testing and AI automation field. Initially, we are witnessing significant strides in automated testing itself, powered largely by the capabilities of AI. To give you a clearer picture, certain AI testing tools are already capable of authoring auto-code based solely on the given requirements. This advancement indicates a significant phase where automated testing is experiencing an evolution, facilitating not just efficiency but also innovation in testing strategies.
AI is increasingly playing a key role in enhancing automated testing methods. It’s helping to fill gaps where traditional automated testing doesn’t suffice, making tasks in Quality Assurance smoother and more efficient. AI achieves this by creating and adjusting test plans, scrutinizing test assessments to ensure requirements are met, creating test cases and identifying defects.
Moreover, AI aids in sorting and prioritising tests, a capability now supported by many independent tools. Consequently, AI is gradually bringing together these elements, allowing for comprehensive data analysis while reducing the need for human intervention. This lets humans focus more on crucial decision-making tasks.
The transformation brought by AI doesn’t just alter how operations are handled; it also boosts productivity and efficiency. As AI testing software evolves, it can better address the intricate and changing needs of software ecosystems. Expect a big drop in the time spent on repetitive tasks, leading to smoother workflows. Additionally, decision-making will be easier and more accurate, thanks to deep analysis of data patterns.
Conclusion
AI solutions elevate QA to a scale that humans canāt achieve. Even if artificial intelligence is still not much of an intelligence, there are a number of exciting ways to apply it in testing. It is all about knowing what you need and picking the right tool.
Modern test management with a forward-looking AI roadmap